PROJECTS
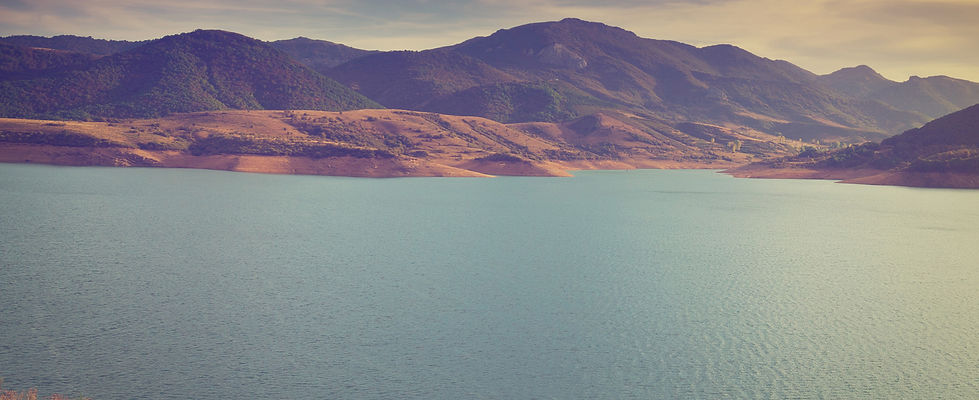
“The shift to a cleaner energy economy won't happen overnight, and it will require tough choices along the way. But the debate is settled. Climate change is a fact."
Barrack Obama
Climate change has been a kind of obsession for me for a few years now, and I have slowly but surely become addicted to watching videos on YouTube that debunk claims made by climate change deniers. For some of the best channels, see here and here. In spite of the vast scientific consensus, there is still a great deal of misunderstanding in the general public concerning whether the earth is even warming [1]. Even our political leaders repeatedly make and defend spurious claims of a “lack of warming for the past 17 years” (e.g. Ted Cruz, [2]), the so-called "warming hiatus." If you simply expand the window and consider data for 100 years, the warming trend is evident, so the 17 year claim seems to be an obvious case of cherry picking. But I started to wonder, how common was it to have a 17-year trend that contradicted the larger 100-year trend?
Towards this aim, I acquired data from the NASA Goddard Institute for Space Studies (GISS) that provides average global (land and ocean) surface temperature for years spanning 1880 – 2014 [3]. To asses spatial patterns in historical temperature, I also acquired monthly global average surface temperatures from Berkeley Earth [4] covering the entire earth surface in a uniform grid with spatial resolution of 1x1 degree latitude/longitude, and spanning the years 1850 – 2013.
To analyze 17 year trends, I computed regression slopes and confidence intervals of NASA-GISS surface temperature and year, for each 17 year interval since 1880 (118 total). To visualize spatial patterns in recent surface temperature increases, I averaged the Berkeley surface data across the intervals corresponding to each of the previous two decades, and looked at their difference on the globe.
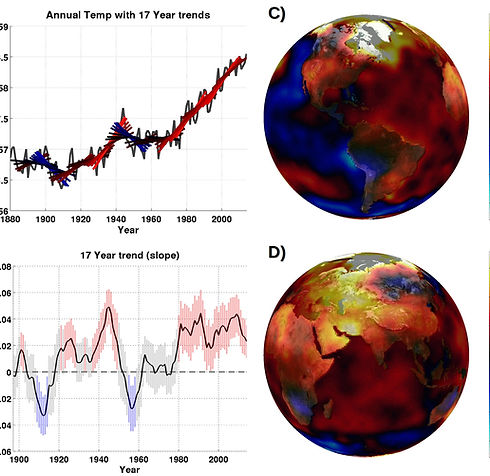
Figure 1 summarizes my findings thus far [5]. Figure 1a shows global average surface temperature by year, along with regression lines for each 17 year interval since 1880. Bright red and blue regression lines indicate strong positive and negative slopes, respectively, with darker gray/black lines indicating flat slopes. Figure 1b depicts the regression slope with confidence intervals indicating significance at p<0.05 (significant positive/negative slopes shown in red/blue respectively, with non-significant slopes shown in gray).
Together, these figures show that, in addition to 2014 being the hottest year ever on record, 17 year slopes have been significantly positive consistently throughout the past three decades. Moreover, 17 year trends with slopes in the range of that currently seen are in no way infrequent since 1880, an interval which has seen global temperatures rise on average almost 2 degrees (F). Despite a large margin for error in assessing significance for intervals so short, it is clear that the “no warming for 17 years” claim can be meaningfully rejected. Figure 2c, and d show spatial patterns of global temperature from the 90's to the 2000's. These figures show that, while recent temperatures have decreased over certain parts of the world (notably the pacific ocean), a far greater proportion of the earth's surface has seen temperature increase, with notable areas in the Arctic and central Asia experiencing increases in excess of 0.015 degrees (F) in a single decade.
Figure 1
Considering how much of the country is still in denial of climate change, I started to wonder how best to prepare our communities at the local, state, and federal level, under the assumption that future temperature increases are no longer entirely preventable.
I wanted to identify the communities most vulnerable, not only to understand how federal and state resources would need to be allocated, or to help inform consumers and businesses relocating homes and facilities, but in the hopes that actually seeing how their home town would be affected would help change the hearts and minds of the American populace.
I acquired fine-scale projected climate-scenarios from the NASA Climate Model Data Service [6]. These data (described in [7]) give projected changes in annual mean temperature and precipitation over the course of the coming century for a grid of latitude and longitude covering the contiguous United States, with extremely fine spatial resolution (~800m x 800m). In addition, I attained data concerning the median yearly household income for ~32,000 U.S. zip codes [8], under the assumption that poorer communities would have fewer resources for combating climate related threats, and would thus be higher priority for the allocation of state and federal resources. From these data I derived 40-year warming and drought related risk indices (denoted Rw, and Rd) for each zip code. Since the median yearly income across zip codes is highly skewed, I defined these indices as:
Rw(zip) = zscore(dW(zip)) – zscore(log(Income(zip)))
Rd(zip) = -zscore(dP(zip)) – zscore(log(Income(zip)))
Where dW and dP are the projected changes in warming and precipitation for each zip code, respectively, which were computed by finding the latitude and longitude on the down sampled NASA grid closest to the geographic center of each zip code (obtained via [9]), and averaging the projected changes over the years 2040 through 2050. The initial negative sign in the formula for Rd indicates that drought risk is associated with decreases in projected precipitation.

Figure 2
Figure 2 [10] shows projected warming and precipitation changes (a, and b), as well as 40-year warming and drought risk indices (c, and d) interpolated across the United states. In all plots, dark red and dark blue indicate maximal and minimal values, respectively. Figure 1 shows that warming will be especially prevalent across the Midwest (2a), but that lower income communities more susceptible to warming risk (2b) are more distributed across the states, with notable clusters in Michigan and the deep South. Additionally, projected precipitation changes and associated drought risk indices indicate that California will be hit hard by changing climate, despite the fact that several Northern California zip codes have much higher than average median incomes.
Although median household income is likely a valid indicator of the local resources available to a community to combat climate change related threats, it is obviously not descriptive enough, and population density is going to be another key consideration. It is my intention to acquire further data concerning local resilience of water, electric, and transportation grids (i.e. robustness to flood/wildfire/severe weather). Additionally, I intend to gather data to asses local industrial resilience, i.e. the proportion of local economy likely to be affected by climate change, either directly (i.e. agriculture, recreation/tourism) or indirectly by national attempts to reduce carbon emissions (energy). I'd like to put together an app that could allow users to simply input their zipcode and see in detail the risk to their hometown.
All of these plans are fairly straightforward, but what I would really like to do is see if there is any way to make use of past historical data to really put a dollar amount on how much these 40-year predictions are going to cost us. So I am setting about gathering international data concerning economic disruptions and humanitarian crises related to climate change which have occurred in the past century, looking for patterns which can be predicted/informed by the Berkeley surface data. Type in your zipcode and see how much it is going to cost in 40 years to keep your town viable. That's the idea. Check back for updates!
References.
[1] http://environment.yale.edu/climate-communication/article/yale-climate-opinion-maps/
[2] http://www.washingtonpost.com/blogs/fact-checker/wp/2015/03/26/ted-cruzs-claim-that- there-has-been-zero-global-warming-in-17-years/
[3] http://data.giss.nasa.gov/gistemp/tabledata_v3/GLB.Ts+dSST.txt
[4] http://berkeleyearth.org/data/
[6] https://cds.nccs.nasa.gov/bioclim/
[7] http://www.nature.com/nclimate/journal/v4/n3/full/nclimate2113.html
[8] http://www.psc.isr.umich.edu/dis/census/Features/tract2zip/
[9] https://www.gaslampmedia.com/download-zip-code-latitude-longitude-city-state-county-csv/